By Dan Kraemer
If 2023 was the year of generative AI experimentation, 2024 is the year of implementation.
Companies in every sector know they need to add generative AI applications and frameworks to their tech stack this year — or get left behind. If the idea of becoming an AI-first company feels daunting, you are not alone.
Even the largest enterprises are still figuring out how to weave generative AI into their everyday businesses.
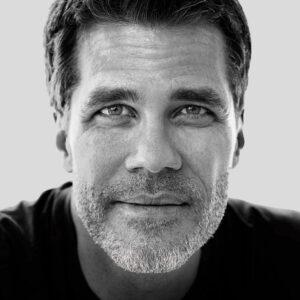
Where do you start when there are so many options?
One of the best ways is to build a “GenAI innovation layer” into your tech stack. You don’t need a large team of data scientists or engineers to do this; there are steps that even companies with small tech teams can take right now.
First off, what is a GenAI innovation layer? Quite simply, it’s a way to weave generative AI into your everyday business activities to unleash new creative workflows, spur innovation projects, and get work done faster and smarter.
Below I will walk through a five-step process to build a GenAI innovation layer that can help business and creative teams design new innovation products and boost creative output.
Step one: Build the right team
To create an AI innovation layer, you need the right team. That does not necessarily mean you need a large team of data scientists and software engineers, but you do need a few key people to lead the project.
The most important thing to define at the outset is how the project will be structured, who will have access to critical data, and how that data will be secured. And it goes without saying that everyone across the organization should be “in your corner” about the importance of building an AI innovation layer.
Building an AI innovation layer should not be a side project, but instead an integral business process for 2024.
Step two: Organize your data
To weave AI throughout your tech stack, first you need to ensure your data is in tip-top order. The easiest way to organize your structured data so it’s accessible to an AI layer is to deploy index-builder tools like Chroma DB, Pinecone or LlamaIndex to create vector databases of your content.
Your structured data can include customer data, video transcripts, documents, PDFs, presentations, spreadsheets and more. The beauty of these systems is that they allow users to handle unstructured data such as interview transcripts, customer feedback and process documents extremely well.
There is some art to collecting, processing and making sure that sensitive information is redacted or secure in these systems. Some tools like LlamaIndex do some of this heavy lifting for you, while other database tools like Elastic can also provide security insights into how your database is being used.
Developers have lots of options here, and your team needs to select which best meet the scale, security and architecture requirements of your project. When you’ve organized all of your data into a vector database, you end up with a “digital twin” that is a full mirror of all your data, one that is now accessible to any of your AI systems.
Step three: Build structures that allow AI to query your data
Now that your data is in order, you need to find patterns within it. You can do this by building custom AI agents to query it. These agents should be accessible through simple-to-use interfaces, such as a chat bar, that are integrated directly into peoples’ daily workflows.
Creating these prompts should happen in two parts. First, decide which personas you want the agent to take on. You could have your agent speak like a management consultant who is an expert in consumer-led design, for example.Then you need to specify what you want the agent to do and the format to respond. The key here is doing the research within your organization to understand which formats are going to best meet the needs of your teams. We suggest testing and iterating on these internal agent prompts with your target users. There is no perfect way to write these internal agent prompts, but there are common prompting methods anyone can use. Such as “walk me through your thinking and give me three examples with X and Y measurable metrics for each, presented in a table.”
Step four: Experiment with multi-agent prompting
Finally, you are ready to ask your AI agent a question. The more context you can provide in your question the better.
Once you have built multiple prompts that are integrated into daily workflows, you can start training them to work together with multi-agent systems. This is where you string together multiple AI agents with different, but intersecting roles.
Together, they can complete a task better than a single agent could. There are several examples of this application, including from ChatDev, a multi-agent virtual software company.
When building multi-agents, we have seen the best success by taking a human-centered design approach. You should ensure your prompts, on their own and working together as multi-agents, operate with empathetic mindsets and an appreciation for the diversity of their users. You can build prompts with parameters such as “design your answers with empathy, tolerance and clarity into the varied experiences of your users.”
At this stage, you may want to incorporate some sort of model oversight. Adding tools like Openlayer to your stack can give you insight into how well your system is performing for users over time.
Step five: Measure your AI innovation layer
Weaving AI into everything you do is only useful if it helps your company achieve business goals, generate new revenue and better serve your customers. To that end, you need to put a measurement framework in place to ensure your AI innovation layer is delivering true value.
Decide what KPIs to measure — new customer sign-ups, product downloads or employee time saved, for example. You can also use your multi-agent prompts to generate future potential scenarios for products, business outcomes and other measurable business goals. Then, you can measure your actual outcomes against these scenarios by again using your multi-agent prompts to create visualizations.
Generative AI has already upended how people work, think and create, but it has largely been an experimental tool used by individuals. By weaving generative AI into your tech stack, it can become an integral part of everyday creative and business workflows — providing new insights into your company’s data, processes and future strategies.
Dan Kraemer is a founder, thought leader, educator and international speaker on the convergence of design and business strategy. As co-CEO and co-founder of IA Collaborative, he combines a generative design lens with analytical business strategy to help companies such as Airbnb, Allstate, Audi, FedEx, Johnson & Johnson, Nike, Philips, Samsung and Sonos create growth strategies, develop innovation and build new businesses.
Related reading:
Illustration: Dom Guzman
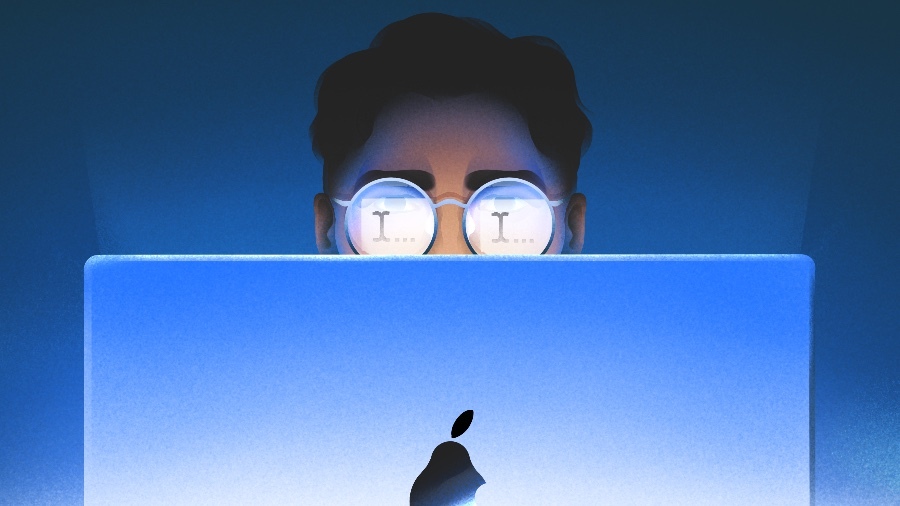