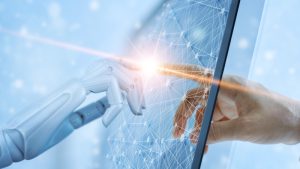
The functionality and complexity of business-oriented AI applications have increased exponentially. Therefore, organizations need new capabilities, such as explainable AI, now more than ever. DevOps tools, security response systems, search technologies, and more have all benefited from AI technology’s progress. Automation and analysis features, in particular, have boosted operational efficiency and performance by tracking and responding to complex or information-dense situations.
However, the ever-growing complexity of AI models introduces a glaring issue: transparency. Many cutting-edge AI models have become so complex in how they decide on an output that even domain experts can’t understand how or why a model makes its decisions. This is often called the black-box problem — and explainable AI aims to address it.
In what follows, we discuss the importance of explainable AI, the challenges of enacting it, and the key components to look for in an AI-powered solution.
What is explainable AI, and why is it essential?
Explainable AI is an aspect of artificial intelligence that aims to make AI more transparent and understandable, resulting in greater trust and confidence from the teams benefitting from the AI. In a perfect world, a robust AI model can perform complex tasks while users observe the decision process and audit any errors or concerns.
The importance of AI understandability is growing irrespective of the application and sector in which an organization operates. For instance, finance and healthcare applications may need to meet regulatory requirements involving AI tool transparency. Safety concerns are at the forefront of autonomous vehicle development, and model understandability is crucial to improving and maintaining functionality in such technology. Therefore, explainable AI is often more than a matter of convenience — it’s a critical part of business operations and industry standards.
As more AI-powered technologies are developed and adopted, more government and industry regulations will be enacted. In the EU, for instance, the EU AI Act is mandating transparency for AI algorithms, though the current scope is limited. Because AI is such a powerful tool, it’s expected to continue to increase in popularity and sophistication, leading to further regulation and explainability requirements.
There’s also concern about bias and dependability in AI models. Generative AI hallucinations have been a popular talking point recently. But AI models have an established history of output bias based on race, gender, and more. Explainable AI tools and practices are important for understanding and weeding out biases like this to improve output accuracy and operational efficiency.
Ultimately, explainable AI is about streamlining and improving an organization’s capabilities. More transparency means a better understanding of the technology being used, better troubleshooting, and more opportunities to fine-tune an organization’s tools.
The current challenges of explainable AI facing organizations
Explainable AI can mean a few different things, so defining the term itself is challenging. To some, it’s a design methodology — a foundational pillar of the AI model development process. Explainable AI is also the name of a set of features or capabilities expected from an AI-based solution, such as decision trees and dashboard components. The term can also point to a way of using an AI tool that upholds the tenets of AI transparency. While all of these are valid examples of explainable AI, its most important role is to promote AI interpretability across a range of applications.
Another limitation of current explainable AI technologies is their effectiveness varies depending on the model. Some models, like deep learning or neural network-based models, are dense and complex, making them difficult to interpret. Decision trees and linear models, on the other hand, are easier to make understandable and transparent due to their more straightforward decision process via dependency mapping.
Explainable AI methodologies are still in the early stages of development. Five years from now, there will be new tools and methods for understanding complex AI models, even as these models continue to grow and evolve. Right now, it’s critical that AI experts and solution providers continue to strive for explainability in AI applications to provide organizations with safe, reliable, and powerful AI tools.
Breaking down the key components of explainable AI
Explainable AI is a broad topic. Therefore, it’s hard to compile a defined list of characteristics for all explainable AI solutions. Some approaches favor certain aspects of the methodology over others, or only apply to certain machine learning models. However, any comprehensive explainable AI approach needs to consider the following components:
- Interpretability. A baseline functionality for interpreting an AI model is necessary. AI predictions, decisions, and other outputs have to be understandable to a human and, at the very least, should be traceable through the model’s decision-making process. The depth of interpretability your organization needs will likely depend on the model you need to make more understandable and your use cases.
- Communication methods. How an explainable AI-oriented offering communicates information is also critical. Strong visualization tools are necessary to fully maximize any explainable AI method’s benefits. Decision trees and dashboards are two common visualization methods that present complex data in an easily readable format. These tools can turn data into actionable insights. Again, the usefulness of various visualization tools depends on the AI model.
- Global vs. local understandability. Finally, global and local explanations have an important distinction. Global explanations are analyses and information that give users insight into how the model is acting as a whole. This can involve showing which portions of data are used during a series of jobs, where automated systems are acting, what they’re doing, and more. Local explanations are insights into an AI model’s individual decisions. These are important if an organization needs to understand an odd or incorrect output or have transparent information on hand for industry regulation reasons.
Looking forward with explainable AI and observability
Explainable AI is a rapidly changing area of AI technology development, though there are already exciting new ways to use AI because of this increase in model explainability.
Dynatrace Davis AI is already in use at large organizations as an example of a robust AI-powered tool utilizing explainable AI methodologies. For more information about how explainable AI and increased observability can improve operations, explore the Dynatrace Perform presentation on how major corporations are using Davis AI to manage a microservices architecture.
The post What is explainable AI? The key to closing the AI confidence gap appeared first on Dynatrace news.