Building on my previous column, I will dive into part deux, going deeper into the application of Generative AI and how we should think about safety and security as a risk management problem. Where do security, privacy and safety intersect? Well, sometimes they are combined into one general overarching function that tries to address everything, and other times they are split into many smaller functions with their own focused teams and expertise. In this article I’ll share my views on how to think about these 3 overlapping categories both as distinct functions as well as how they intersect.
Safety
Safety is the broadest category and it provides the guardrails that define how AI and humans interact with each other. Safety is also often bundled with content moderation in the social networking world. While some of that still holds true, safety has even closer ties to content moderation when Generative AI is involved. This can be anything from text or voice-based information accuracy to physical safety, depending on the modality of the model and its real- world implications and interactions. The US Artificial Intelligence Safety Board, or Open AI and Anthropic safety portals provide good examples of focused safety guidelines, providing specific areas where companies invest in controls and testing. In addition, some companies may focus on safety metrics in comparison to humans doing the same task, such as with healthcare or autonomous vehicles.
The three most critical areas of safety for generative AI currently are:
- Bias and Fairness
- Misinformation and Toxicity
- Societal Impact
To understand the stakes of generative AI safety, imagine AI was asked to solve the classic ethical dilemma of the trolley problem using the criteria above. If you need a refresher: A hypothetical runaway trolley is heading down a track towards five people who are tied up and unable to move. You are standing next to a lever. If you pull the lever, the trolley will switch to a different track where there is one person tied up. What do you do? While this is a purely a thought exercise, the responses to this question clearly fall into the safety realm. Models currently cannot understand emotion or human bonds, and thus fall short in empathy or decision making around empathy. They also lack long term predictive power, mainly because there are not enough compute resources to factor in all past, present and future data. The only way to advance AI safety is to increase human interactions, human values and societal governance to promote a reinforced human feedback loop, much like we do with traditional AI training methods.
Security
Security is the ability to protect the service against misuse that could lead to unauthorized access to systems or data. Some clear examples that fall into security are:
- Vulnerabilities within the codebase
- Multi-step role playing (social engineering for AI)
- Data storage and access
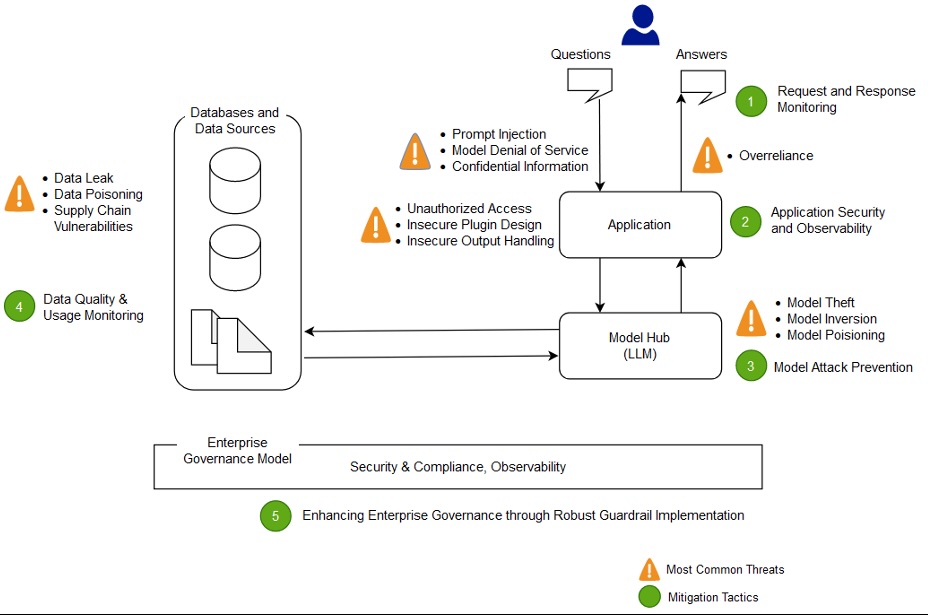
As we see in the diagram, AI security borrows from the same concepts of traditional software development security, including core areas for protecting the data, third party libraries, and the overall development pipeline. Some of the new generative AI specific areas include the attack surface area of unstructured data inputs and complex interactions within the model based on probabilistic measures. In addition, model parameters offer a lucrative target for bad actors to recreate a model that would have cost many millions to train. Prompt security, the field of preventing unauthorized manipulation and misuse of generative AI, is a very well-researched area that will continue to grow and expand. Facebook for example has released two open source models, Llama Guard for prompt safety and Prompt Guard for prompt security. Interestingly, Facebook does not currently offer a privacy tool.
Privacy
Privacy addresses the collection and usage of data. One of the key concerns with AI is the massive amount of data it must have access to in order to serve content well. Microsoft recently suffered massive backlash over a feature that recorded your screen as you used the device, as well as turning on training by default for its users.
Advertisement. Scroll to continue reading.
With privacy risk, you often weigh the regulatory outcomes, publicity, and direct financial damages. Some examples include:
- Data residency and localized processing
- Laws and regulations
- Third party data transfers
Behavioral tracking and social scoring are a major concern in advertising, and now with more powerful models, more data can be stitched together from disparate sources to deanonymize what used to be considered private data. A good paper presented at the Conference on Human Factors in Computing Systems outlines how AI data collection can be used to degrade privacy for all internet users. This is especially important as we think about the supply chains involved for AI, and how more third parties are getting access to data.
Image Credit:Deepfakes, Phrenology, Surveillance, and More! A Taxonomy of AI Privacy Risks
And finally we can tie them all together under a general framework such as with the NIST AI Risk Management Framework. One reason I like this framework in particular is that it provides high-level guidance on managing AI risk, rather than focusing on a single element of the fast-moving technology. It also groups them into very easy-to-understand categories such as Map, Manage, Measure and Govern. Map is the research phase, spending time to understand the AI system in context and create documentation around its usage. Measure is the measurement phase, where quantitative and qualitative measures are established to create internal benchmarks. Manage is used to develop risk treatment strategies to address issues and Govern is the overarching process that ensures risk accountability and ownership.
These principles are taken from other NIST frameworks and have been largely successful in adoption across many private and public sector security programs. The big reason that this framework exists is to ensure that AI systems will be used responsibly. In addition, clear legal guidance, model transparency, model ownership and data usage rules will allow AI to grow and for users to benefit from collectively.
As we understand more about how security, safety and privacy apply to new technology, it’s important to understand the distinction between them. Industry should understand the important nuances of each of these key areas so that we develop ways to mitigate each of the risks, both individually and as a whole.
This column is Part 2 of multi-part series on securing generative AI:
Part 1: Back to the Future, Securing Generative AI
Part 2: Trolley problem, Safety Versus Security of Generative AI
Part 3: Build vs Buy, Red Teaming AI (Stay Tuned)
Part 4: Timeless Compliance (Stay Tuned)