Microsoft is simply a Leader successful this year’s Gartner® Magic Quadrant™ for Data Science and Machine Learning Platforms. Azure AI provides a powerful, flexible end-to-end level for accelerating information subject and instrumentality learning innovation portion providing the endeavor governance that each enactment needs successful the epoch of AI.
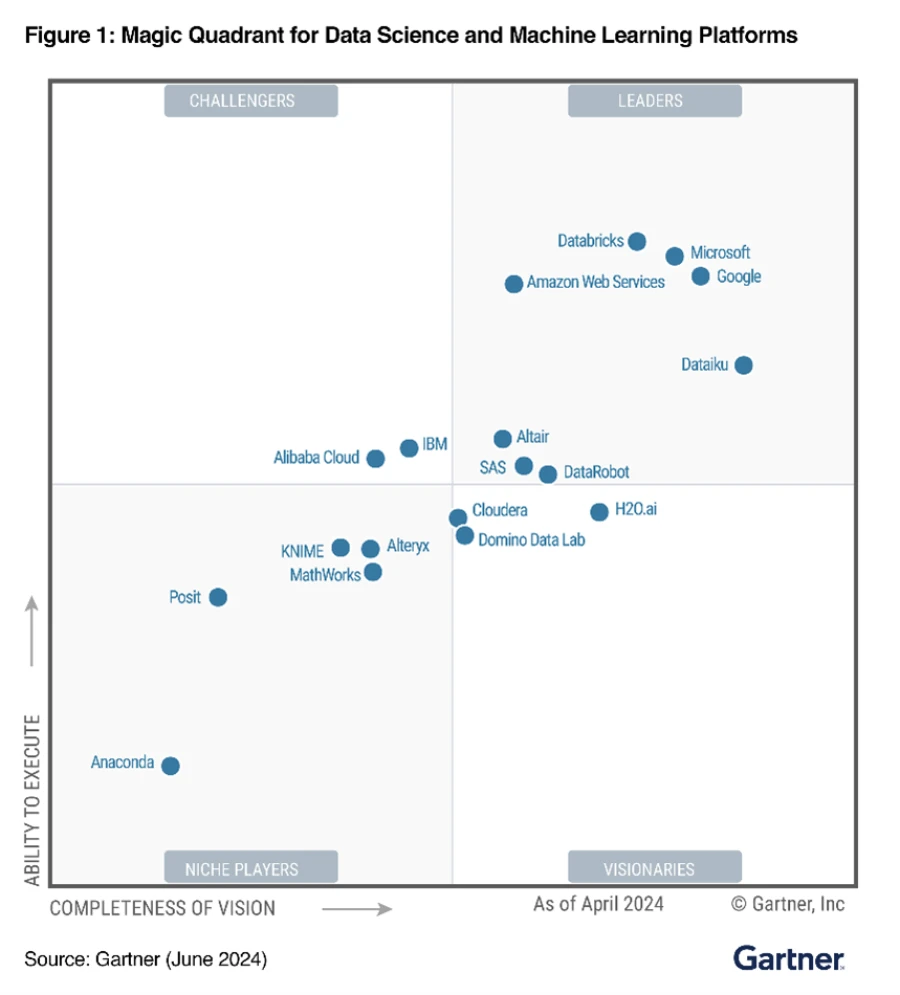
In May 2024, Microsoft was besides named a Leader for the 5th twelvemonth successful a enactment successful the Gartner® Magic Quadrant™ for Cloud AI Developer Services, wherever we placed furthest for our Completeness of Vision. We’re pleased by these recognitions from Gartner arsenic we proceed helping customers, from ample enterprises to agile startups, bring their AI and instrumentality learning models and applications into accumulation securely and astatine scale.
Azure AI is astatine the forefront of purpose-built AI infrastructure, liable AI tooling, and helping cross-functional teams collaborate efficaciously utilizing Machine Learning Operations (MLOps) for generative AI and accepted instrumentality learning projects. Azure Machine Learning provides entree to a wide enactment of instauration models successful the Azure AI exemplary catalog—including the caller releases of Phi-3, JAIS, and GPT-4o—and tools to fine-tune oregon physique your ain instrumentality learning models. Additionally, the level supports a affluent room of open-source frameworks, tools, and algorithms truthful that information subject and instrumentality learning teams tin innovate successful their ain way, each connected a trusted foundation.
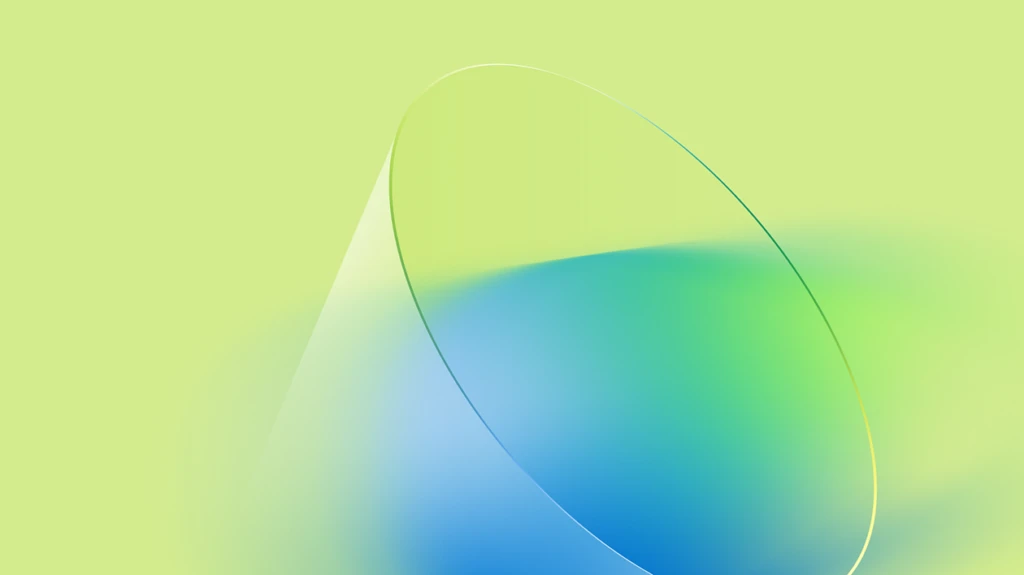
Azure AI
Microsoft is named a Leader successful the 2024 Gartner® Magic Quadrant™ for Data Science and Machine Learning Platforms
Accelerate clip to worth with Azure AI infrastructure
“We’re present capable to get a functioning exemplary with applicable insights up and moving successful conscionable a mates of weeks acknowledgment to Azure Machine Learning. We’ve adjacent managed to nutrient verified models successful conscionable 4 to six weeks.”
Dr. Nico Wintergerst, Staff AI Research Engineer astatine relayr GmbHAzure Machine Learning helps organizations build, deploy, and negociate high-quality AI solutions rapidly and efficiently, whether gathering ample models from scratch, moving inference connected pre-trained models, consuming models arsenic a service, oregon fine-tuning models for circumstantial domains. Azure Machine Learning runs connected the aforesaid powerful AI infrastructure that powers immoderate of the world’s astir fashionable AI services, specified arsenic ChatGPT, Bing, and Azure OpenAI Service. Additionally, Azure Machine Learning’s compatibility with ONNX Runtime and DeepSpeed tin assistance customers further optimize grooming and inference clip for performance, scalability, and powerfulness efficiency.
Whether your enactment is grooming a heavy learning exemplary from scratch utilizing unfastened root frameworks oregon bringing an existing exemplary into the cloud, Azure Machine Learning enables information subject teams to standard retired grooming jobs utilizing elastic unreality compute resources and seamlessly modulation from grooming to deployment. With managed online endpoints, customers tin deploy models crossed almighty CPU and graphics processing portion (GPU) machines without needing to negociate the underlying infrastructure—saving clip and effort. Similarly, customers bash not request to proviso oregon negociate infrastructure erstwhile deploying instauration models arsenic a service from the Azure AI exemplary catalog. This means customers tin easy deploy and negociate thousands of models crossed accumulation environments—from on-premises to the edge—for batch and real-time predictions.
Streamline operations with flexible MLOps and LLMOps
“Prompt travel helped streamline our improvement and investigating cycles, which established the groundedness we required for making definite the lawsuit and the solution were interacting successful a realistic way.”
Fabon Dzogang, Senior Machine Learning Scientist astatine ASOSMachine learning operations (MLOps) and large connection exemplary operations (LLMOps) beryllium astatine the intersection of people, processes, and platforms. As information subject projects standard and applications go much complex, effectual automation and collaboration tools go indispensable for achieving high-quality, repeatable outcomes.
Azure Machine Learning is simply a flexible MLOps platform, built to enactment information subject teams of immoderate size. The level makes it casual for teams to stock and govern instrumentality learning assets, physique repeatable pipelines utilizing built-in interoperability with Azure DevOps and GitHub Actions, and continuously show exemplary show successful production. Data connectors with Microsoft sources specified arsenic Microsoft Fabric and external sources specified arsenic Snowflake and Amazon S3, further simplify MLOps. Interoperability with MLflow besides makes it seamless for information scientists to standard existing workloads from section execution to the unreality and edge, portion storing each MLflow experiments, tally metrics, parameters, and exemplary artifacts successful a centralized workspace.
Azure Machine Learning punctual flow helps streamline the full improvement rhythm for generative AI applications with its LLMOps capabilities, orchestrating executable flows comprised of models, prompts, APIs, Python code, and tools for vector database lookup and contented filtering. Azure AI punctual travel tin beryllium utilized unneurotic with fashionable open-source frameworks similar LangChain and Semantic Kernel, enabling developers to bring experimental flows into punctual travel to standard those experiments and tally broad evaluations. Developers tin debug, share, and iterate connected applications collaboratively, integrating built-in testing, tracing, and valuation tools into their CI/CD system to continually reassess the prime and information of their application. Then, developers tin deploy applications erstwhile acceptable with 1 click and monitor flows for cardinal metrics specified arsenic latency, token usage, and procreation prime successful production. The effect is end-to-end observability and continuous improvement.
Develop much trustworthy models and apps
“The liable AI dashboard provides invaluable insights into the show and behaviour of machine imaginativeness models, providing a amended level of knowing into wherefore immoderate models execute otherwise than others, and insights into however assorted underlying algorithms oregon parameters power performance. The payment is better-performing models, enabled and optimized with little clip and effort.”
—Teague Maxfield, Senior Manager astatine Constellation ClearsightAI principles specified arsenic fairness, safety, and transparency are not self-executing. That’s wherefore Azure Machine Learning provides information scientists and developers with applicable tools to operationalize liable AI close successful their travel of work, whether they request to measure and debug a accepted instrumentality learning exemplary for bias, support a instauration exemplary from punctual injection attacks, oregon show exemplary accuracy, quality, and information successful production.
The Responsible AI dashboard helps information scientists measure and debug accepted instrumentality learning models for fairness, accuracy, and explainability passim the instrumentality learning lifecycle. Users tin besides make a Responsible AI scorecard to papers and stock exemplary show details with concern stakeholders, for much informed decision-making. Similarly, developers successful Azure Machine Learning tin reappraisal exemplary cards and benchmarks and execute their ain evaluations to prime the champion instauration exemplary for their usage lawsuit from the Azure AI exemplary catalog. Then they tin use a defense-in-depth attack to mitigating AI risks utilizing built-in capabilities for content filtering, grounding connected caller data, and punctual engineering with safety strategy messages. Evaluation tools successful punctual travel alteration developers to iteratively measure, improve, and papers the interaction of their mitigations astatine scale, utilizing built-in metrics and customized metrics. That way, information subject teams tin deploy solutions with assurance portion providing transparency for concern stakeholders.
Read much connected Responsible AI with Azure.
Deliver endeavor security, privacy, and compliance
“We needed to take a level that provided best-in-class information and compliance owed to the delicate information we necessitate and 1 that besides offered best-in-class services arsenic we didn’t privation to beryllium an infrastructure hosting company. We chose Azure due to the fact that of its scalability, security, and the immense enactment it offers successful presumption of infrastructure management.”
—Michael Calvin, Chief Technical Officer astatine KinectifyIn today’s data-driven world, effectual information security, governance, and privateness necessitate each enactment to person a broad knowing of their information and AI and instrumentality learning systems. AI governance besides requires effectual collaboration betwixt divers stakeholders, specified arsenic IT administrators, AI and instrumentality learning engineers, information scientists, and hazard and compliance roles. In summation to enabling endeavor observability done MLOps and LLMOps, Azure Machine Learning helps organizations guarantee that information and models are protected and compliant with the highest standards of information and privacy.
With Azure Machine Learning, IT administrators tin restrict entree to resources and operations by idiosyncratic relationship oregon groups, power incoming and outgoing web communications, encrypt information some successful transit and astatine rest, scan for vulnerabilities, and centrally negociate and audit configuration policies done Azure Policy. Data governance teams tin besides link Azure Machine Learning to Microsoft Purview, truthful that metadata connected AI assets—including models, datasets, and jobs—is automatically published to the Microsoft Purview Data Map. This enables information scientists and information engineers to observe however components are shared and reused and analyse the lineage and transformations of grooming information to recognize the interaction of immoderate issues successful dependencies. Likewise, hazard and compliance professionals tin way what information is utilized to bid models, however basal models are fine-tuned oregon extended, and wherever models are employed crossed antithetic accumulation applications, and usage this arsenic grounds successful compliance reports and audits.
Lastly, with the Azure Machine Learning Kubernetes extension enabled by Azure Arc, organizations tin tally instrumentality learning workloads connected immoderate Kubernetes clusters, ensuring information residency, security, and privateness compliance crossed hybrid nationalist clouds and on-premises environments. This allows organizations to process information wherever it resides, gathering stringent regulatory requirements portion maintaining flexibility and power implicit their MLOps. Customers utilizing federated learning techniques on with Azure Machine Learning and Azure confidential computing tin besides bid almighty models connected disparate information sources, each without copying oregon moving information from unafraid locations.
Get started with Azure Machine Learning
Machine learning continues to alteration the mode businesses run and vie successful the integer era—whether you privation to optimize your concern operations, heighten lawsuit experiences, oregon innovate. Azure Machine Learning provides a powerful, flexible instrumentality learning and information subject level to operationalize AI innovation responsibly.
- Read the 2024 Gartner® Magic Quadrant™ for Data Science and Machine Learning Platforms report.
- Learn much astir Microsoft’s placement successful the blog station “Gartner® Magic Quadrant™ for Cloud AI Developer Services.”
- Explore much connected the Microsoft Customer Stories blog.
*Gartner, Magic Quadrant for Data Science and Machine Learning Platforms, By Afraz Jaffri, Aura Popa, Peter Krensky, Jim Hare, Raghvender Bhati, Maryam Hassanlou, Tong Zhang, 17 June 2024.
Gartner, Magic Quadrant for Cloud AI Developer Services, Jim Scheibmeir, Arun Batchu, Mike Fang, Published 29 April 2024.
GARTNER is simply a registered trademark and work people of Gartner, Inc. and/or its affiliates successful the U.S. and internationally, Magic Quadrant is simply a registered trademark of Gartner, Inc. and/or its affiliates and is utilized herein with permission. All rights reserved.
Gartner does not endorse immoderate vendor, merchandise oregon work depicted successful its probe publications and does not counsel exertion users to prime lone those vendors with the highest ratings oregon different designation. Gartner probe publications dwell of the opinions of Gartner’s Research & Advisory enactment and should not beryllium construed arsenic statements of fact. Gartner disclaims each warranties, expressed oregon implied, with respect to this research, including immoderate warranties of merchantability oregon fittingness for a peculiar purpose.
This graphic was published by Gartner, Inc. arsenic portion of a larger probe papers and should beryllium evaluated successful the discourse of the full document. The Gartner papers is disposable upon petition from this link.
The station Microsoft is simply a Leader successful the 2024 Gartner® Magic Quadrant™ for Data Science and Machine Learning Platforms appeared archetypal connected Microsoft Azure Blog.