Generative AI continues to amaze users at organizations around the world. From helping marketers workshop ideas and creative campaigns, to recommending coding advice to developers, to assisting analysts with market research, the technology has captivated users with its ability to synthesize information and generate answers to questions.
But the arrival of generative AI hasn’t been without its challenges.
Though foundation models that power generative AI develop a vast “world knowledge” during training, they're only as up-to-date as their training data, and they can lack access to all the data sources pertinent to enterprise use cases. To adopt generative AI at full speed, businesses need to ground foundation model responses in enterprises systems and fresh data, to ensure the most accurate and complete responses.
For instance, by grounding foundation model responses in ERP systems, businesses can create AI agents that provide accurate shipping predictions, and by grounding in documentation and manuals, they can deliver more helpful answers to product questions and for troubleshooting.
Similarly, research can be accelerated by grounding in analyst reports and studies, compliance can be strengthened by connecting foundation models to contracts, and employee training and onboarding can be improved by rooting agents in internal documents, knowledge bases, and HR systems.
Essentially, the more easily businesses can ground foundation models in their data, the more powerful their use cases can become.
At Google Cloud, we call this “enterprise truth” — the approach to grounding a foundation model in web information; enterprise data like databases and data warehouses; enterprise applications like ERP, CRM, and HR systems; and other sources of relevant information. Grounding in enterprise truth significantly improves the completeness and accuracy of responses, unlocking unique use cases across the business and laying the groundwork for the next generation of AI agents.
Let’s explore how we do it!
Grounding generative AI with Google Search and enterprise data
Generative AI models know the most probable response, which isn’t the same as being able to cite facts. This is why we’ve built — and continue to build — a variety of ways to help ensure each organization is able to ground its foundation models in the truth relevant to its use case.
Google Search is one of the world's most trusted sources of factual and up-to-date information. Ground with Google Search expands and enhances the model’s access to fresh, high-quality information, significantly improving the completeness and accuracy of responses.
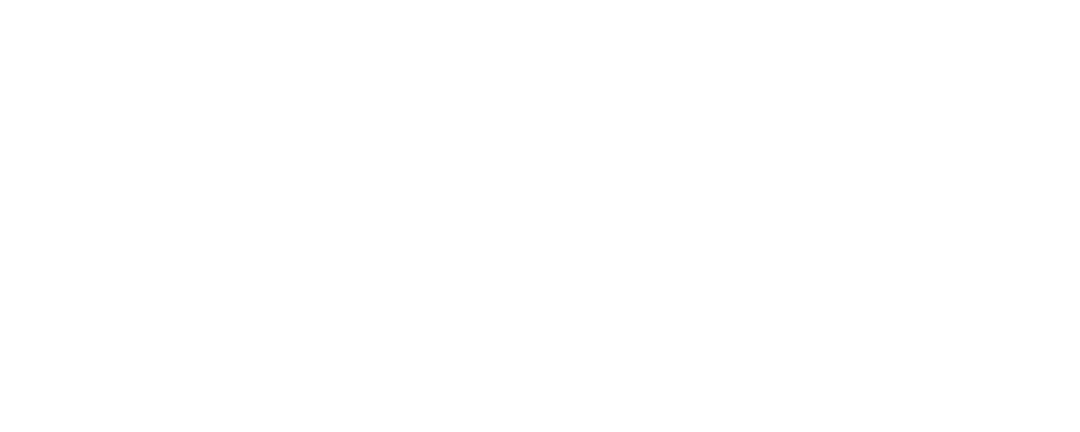
Today, we are announcing the preview of Ground with Google Search in Vertex AI. Businesses can now augment Gemini models with Google Search grounding, and can easily integrate the enhanced model into their AI agents.
When it comes to enterprise data, we offer multiple ways for businesses to ground model responses in enterprise data sources by leveraging retrieval augmented generation, or RAG. RAG helps improve the accuracy of model outputs by using vectors and embeddings to gather facts from relevant data sources.
Vertex AI includes not only a Google-quality, out-of-box RAG solution, but also a variety of component APIs for building bespoke retrieval, ranking, and document processing systems that enable enterprises to easily ground foundation models in their own data.
For organizations that need embeddings-based information retrieval, Vertex AI offers powerful vector search capabilities. Today, to enhance vector search, we are excited to announce the preview of our hybrid search feature, which integrates vector-based and keyword-based search techniques to ensure relevant and accurate responses for users.
Besides these, customers can connect models to Google databases like AlloyDB and BigQuery for the contextual retrieval of operational data and analytics like purchase preferences, rewards, basket analysis, interaction history, and more. To enable actions and transactions, we provide a host of data connectors to help businesses connect their models to enterprise applications like Workday, Salesforce, ServiceNow, Hadoop, Confluence, and JIRA to access the latest data on customer interactions and internal knowledge updates like issue tracking, program management, and employee records.
With a comprehensive approach to grounding that covers web search, enterprise data, and third-party enterprise applications, businesses can ensure that their models will deliver enterprise truth – wherever it is hosted.
How more sources of truth create more value
Let’s walk through an example to show how grounding lets organizations integrate sources of enterprise truth and create more helpful AI agents.
Suppose an athletic brand wants to create an AI agent to help customers find and purchase shoes.
If the company just puts an interface atop a foundation model API, they won’t accomplish much. The resulting app would be able to discuss shoes generally, based on its training knowledge, but it wouldn’t have particular expertise in the brand’s shoes or any awareness of new footwear trends that emerged after its training cutoff date.
With grounding in Google Search, the shoe brand’s app can become much more functional, able to search the web for fresh information. However, it wouldn’t have insight into the brand’s internal data such as product information, inventory levels, and manufacturing timelines, nor would it be able to call functions for transactions — so the shoe company would still be dealing with a basic and rather limited agent.
To cross this chasm, the company also needs to connect its gen AI models to enterprise data sources via RAG mechanisms, so the agent can ground its advice in the specificity and factuality of internal documents and databases.
Imagine an advanced, proactive version of the shoe-recommending agent, with access to the full spectrum of aforementioned search, databases, and analytics. It would be able to observe patterns like the customer’s last several purchases all having green stripes. It would remember the earlier chat in which the customer said they dislike shoes that squeak on hardwood floors, and then go about reviewing customer reviews to purge squeakiness from its recommendations. It would also generate tables on the fly so the customer can more easily compare options, and it would know up-to-date inventory and shipping information to help execute transactions. With the right grounding in enterprise truth, the sky's the limit — and so is the value the agent can create.
Enterprise truth: fueling gen AI innovation across businesses
Generative AI adoption isn’t just about access to capable models. It’s also about grounding foundation models in first-party data and high-quality external sources — and using these connections to steer model behavior, creating more accurate, relevant, and factual generative AI experiences for businesses to offer their customers, partners, and employees.
With access to high-quality and relevant data, models can power experiences that move beyond traditional passive applications, giving rise to the next generation of AI agents grounded in enterprise truth. That’s the future we are rapidly moving towards. Backed by our commitment to this journey with our customers, we’re excited to help make the outputs of today’s agents factual, relevant, and actionable.
To learn more about Google Cloud’s AI news at Next, check out our Vertex AI Agent Builder announcement.