Azure empowers intelligent services similar Microsoft Copilot, Bing, and Azure OpenAI Service that person captured our imaginativeness successful caller days. These services, facilitating assorted applications similar Microsoft Office 365, chatbots, and hunt engines with generative AI, beryllium their magic to ample connection models (LLMs). While the latest LLMs are transcendental, bringing a generational alteration successful however we use artificial quality successful our regular lives and crushed astir its evolution, we person simply scratched the surface. Creating much capable, fair, foundational LLMs that devour and contiguous accusation much accurately is necessary.
How Microsoft maximizes the powerfulness of LLMs
However, creating caller LLMs oregon improving the accuracy of existing ones is nary casual feat. To make and bid improved versions of LLMs, supercomputers with monolithic computational capabilities are required. It is paramount that some the hardware and bundle successful these supercomputers are utilized efficiently astatine scale, not leaving show connected the table. This is wherever the sheer standard of the supercomputing infrastructure successful Azure unreality shines and mounting a caller standard grounds successful LLM grooming matters.
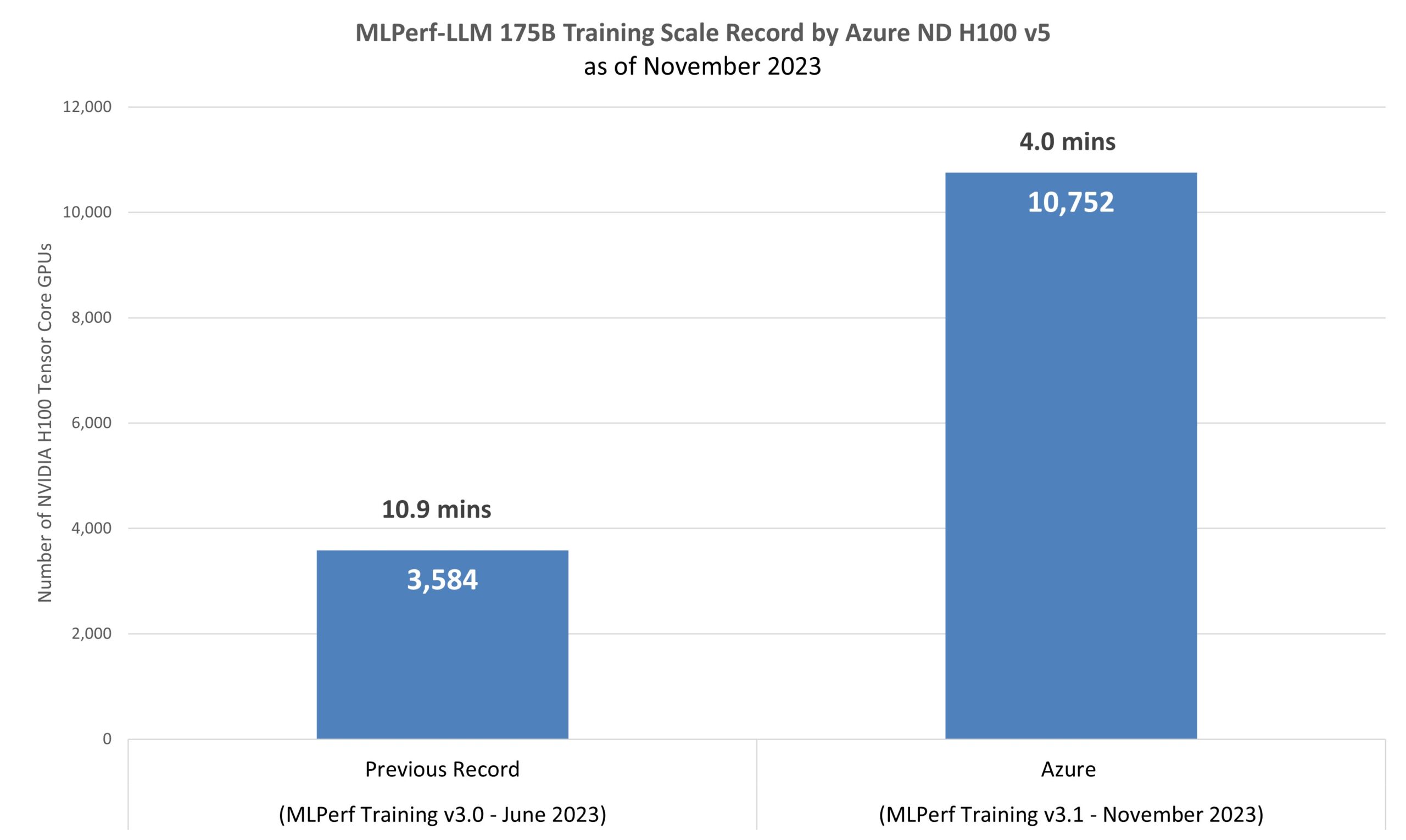
Customers request reliable and performant infrastructure to bring the astir blase AI usage cases to marketplace successful grounds time. Our nonsubjective is to physique state-of-the-art infrastructure and conscionable these demands. The latest MLPerf™ 3.1 Training results1 are a testament to our unwavering committedness to gathering high-quality and high-performance systems successful the unreality to execute unparalleled ratio successful grooming LLMs astatine scale. The thought present is to usage monolithic workloads to accent each constituent of the strategy and accelerate our physique process to execute precocious quality.
The GPT-3 LLM exemplary and its 175 cardinal parameters were trained to completion successful 4 minutes connected 1,344 ND H100 v5 virtual machines (VMs), which correspond 10,752 NVIDIA H100 Tensor Core GPUs, connected by the NVIDIA Quantum-2 InfiniBand networking level (as shown successful Figure 1). This grooming workload uses adjacent to real-world datasets and restarts from 2.4 terabytes of checkpoints acting intimately a accumulation LLM grooming scenario. The workload stresses the H100 GPUs Tensor Cores, direct-attached Non-Volatile Memory Express disks, and the NVLink interconnect that provides accelerated connection to the high-bandwidth representation successful the GPUs and cross-node 400Gb/s InfiniBand fabric.
“Azure’s submission, the largest successful the past of MLPerf Training, demonstrates the bonzer advancement we person made successful optimizing the standard of training. MLCommons’ benchmarks showcase the prowess of modern AI infrastructure and software, underlining the continuous advancements that person been achieved, yet propelling america toward adjacent much almighty and businesslike AI systems.”—David Kanter, Executive Director of MLCommons
Microsoft’s commitment to performance
In March 2023, Microsoft introduced the ND H100 v5-series which completed grooming a 350 cardinal parameter Bidirectional Encoder Representations from Transformers (BERT) connection exemplary successful 5.4 minutes, beating our existing record. This resulted successful a 4 times betterment successful clip to bid BERT wrong conscionable 18 months, highlighting our continuous endeavor to bring the champion show to our users.
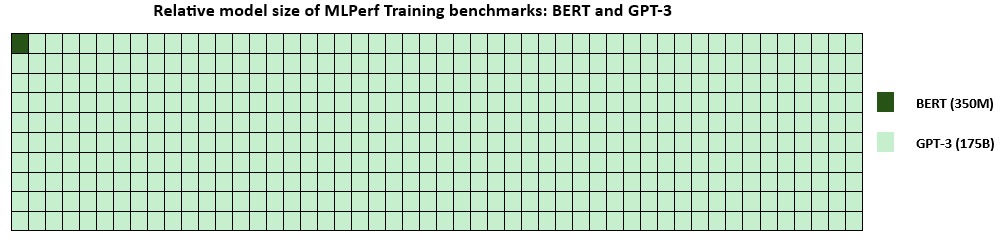
Today’s results are with GPT-3, a ample connection exemplary successful the MLPerf Training benchmarking suite, featuring 175 cardinal parameters, a singular 500 times larger than the antecedently benchmarked BERT exemplary (figure 2). The latest grooming clip from Azure reached a 2.7x betterment compared to the erstwhile grounds from MLPerf Training v3.0. The v3.1 submission underscores the quality to alteration grooming clip and outgo by optimizing a exemplary that accurately represents existent AI workloads.
The powerfulness of virtualization
NVIDIA’s submission to the MLPerf Training v3.1 LLM benchmark connected 10,752 NVIDIA H100 Tensor Core GPUs achieved a grooming clip of 3.92 minutes. This amounts to conscionable a 2 percent summation successful the grooming clip successful Azure VMs compared to the NVIDIA bare-metal submission, which has the best-in-class show of virtual machines crossed each offerings of HPC instances successful the unreality (figure 3).

The latest results successful AI Inferencing connected Azure ND H100 v5 VMs amusement enactment results arsenic well, arsenic shown successful MLPerf Inference v3.1. The ND H100 v5-series delivered 0.99x-1.05x comparative show compared to the bare-metal submissions connected the aforesaid NVIDIA H100 Tensor Core GPUs (figure 4), echoing the ratio of virtual machines.
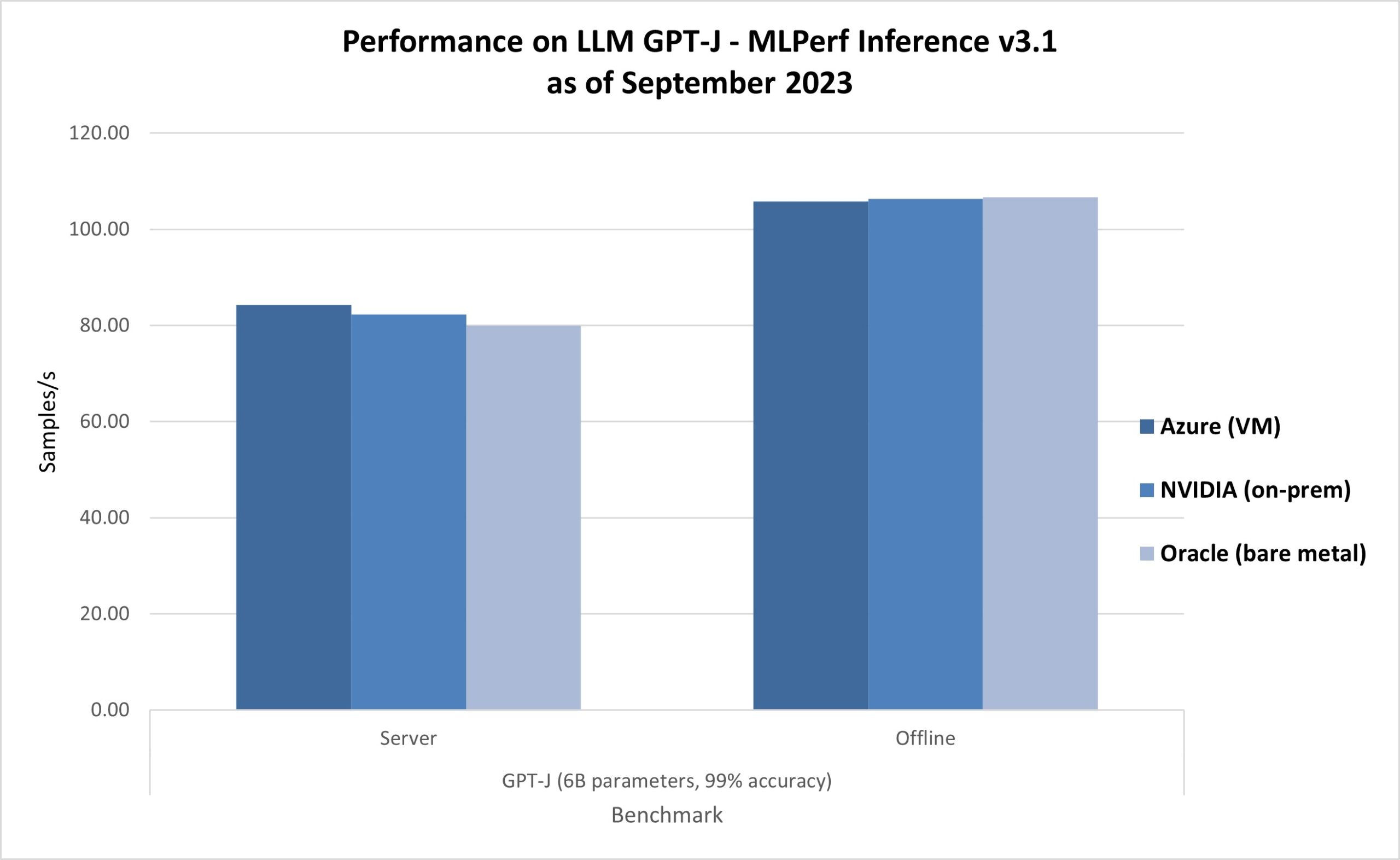
In conclusion, created for performance, scalability, and adaptability, the Azure ND H100 v5-series offers exceptional throughput and minimal latency for some grooming and inferencing tasks successful the unreality and offers the highest prime infrastructure for AI.
Learn more astir Azure AI Infrastructure
References
- MLCommons® is an unfastened engineering consortium of AI leaders from academia, probe labs, and industry. They physique just and utile benchmarks that supply unbiased evaluations of grooming and inference show for hardware, software, and services—all conducted nether prescribed conditions. MLPerf™ Training benchmarks dwell of real-world compute-intensive AI workloads to champion simulate customer’s needs. Tests are transparent and objective, truthful exertion decision-makers tin trust connected the results to marque informed buying decisions.
The station Azure sets a standard grounds successful ample connection exemplary training appeared archetypal connected Microsoft Azure Blog.