In today's data-driven world, organizations face the challenge of managing vast amounts of data while ensuring its security, accessibility, and compliance. For Box.Inc, a global leader in Cloud Content Management, implementing an advanced data catalog solution became crucial to streamline our Data Platform operations. By adopting Google Cloud Dataplex, a capability also within BigQuery, as our go-to tool for enhanced data governance, discovery and observability, Box.Inc successfully transformed our approach towards managing complex analytics use cases to drive product innovation and growth.
Our Data Platform is built on a multi-tenant model grounded in data mesh architecture. Data mesh decentralizes data ownership to teams with the greatest contextual understanding and gives business domains self-serve data platforms and federated governance, allowing them to model, develop, deploy, and operate data services independently. This ensures agile decision-making and efficient data utilization.
Handling billions of files and serving millions of users globally, our Data Platform that’s built on Google Cloud's BigQuery as Data Lake solution, makes it easier for us to process hundreds of thousands of events per second and manages petabyte-scale storage demands with its serverless architecture. Additionally, it manages thousands of query jobs daily, leveraging BigQuery’s massive parallel processing capabilities to process large datasets across various teams within the organization. Balancing this scale with effective infrastructure management is essential to sustain our profitable growth. To plan ahead, we aim to unlock the potential of predictive and prescriptive analytics. By augmenting our Data Platform's capabilities, teams can tackle complex analytical challenges, and drive innovative products that cater to our complex internal and external analytical needs.
Challenges faced by growing business operations
As Box.Inc continued to expand globally, with millions of users relying on our data platform services every day, we encountered several challenges.
-
Data discovery: Product analysts, data scientists, and ML engineers struggled with the time-consuming (multiple days to weeks) processes involved in discovering, retrieving and understanding relevant datasets. Teams didn’t always understand where to find data sourced from a particular product or service, or who could give them access to data, or how existing data was structured.
-
Data observability: Data engineers also found it challenging to monitor data pipelines for debugging purposes, resulting in prolonged data downtime and resolution times (up to a few weeks), impacting productivity significantly.
-
Data lineage: The lack of end-to-end visibility and traceability of data pipelines for data engineers and software developers prevented them from proactively detecting and resolving data issues. It would take them days to weeks to perform impact and root cause analysis.
-
Data governance and security: It’s hard to govern fine-grained data access control over sensitive data to comply with regulations like GDPR, especially amidst extreme growth in volume, variety, and velocity. With a lack of appropriate tools, the Box.Inc Security team faced difficulties identifying, classifying, and protecting sensitive customer information; nor was it easy to find out who could approve data access for production systems.
To address these challenges, we turned to Dataplex, a powerful data governance solution that offers robust capabilities for metadata management and data discovery.
Leveraging Dataplex, we embarked on a transformative journey to enhance our Data Platform by enhancing developer efficiency while tightening security policies across all regions. Dataplex serves as our central data catalog, providing data discovery, lineage tracking, and governance capabilities.
Leveraging Dataplex
Dataplex brings a wide range of capabilities to our practice: Data discovery, data lineage, data observability, data governance, and security for compliance. Let’s take a look at each of these.
1. Streamlined data discovery using metadata tags
Dataplex metadata tags, alongside tag templates, empowered product and business analysts, data scientists, and other stakeholders to discover and utilize specific data more easily by reading operational and business metadata tags associated with each dataset. These standardized metadata frameworks and tag templates facilitated faster insights generation, dashboard creation, and report development, enabling quicker decision-making processes throughout Box.Inc.
Here is the high-level architecture that automates updating custom tag values in Dataplex.
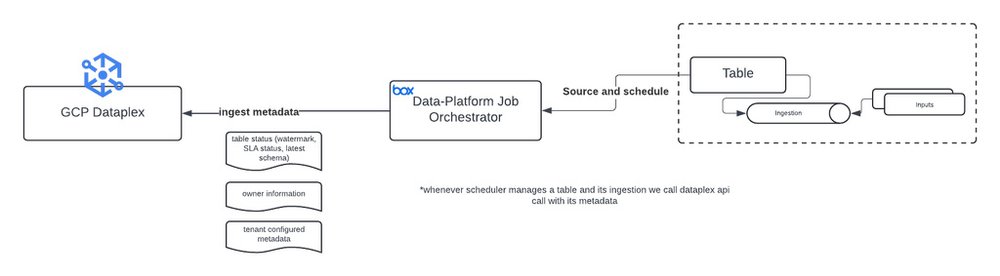
These are examples of public resource-level tags that are created to manage the operational and business metadata to aid in data discovery, such as resource owner, table ingestion, etc.
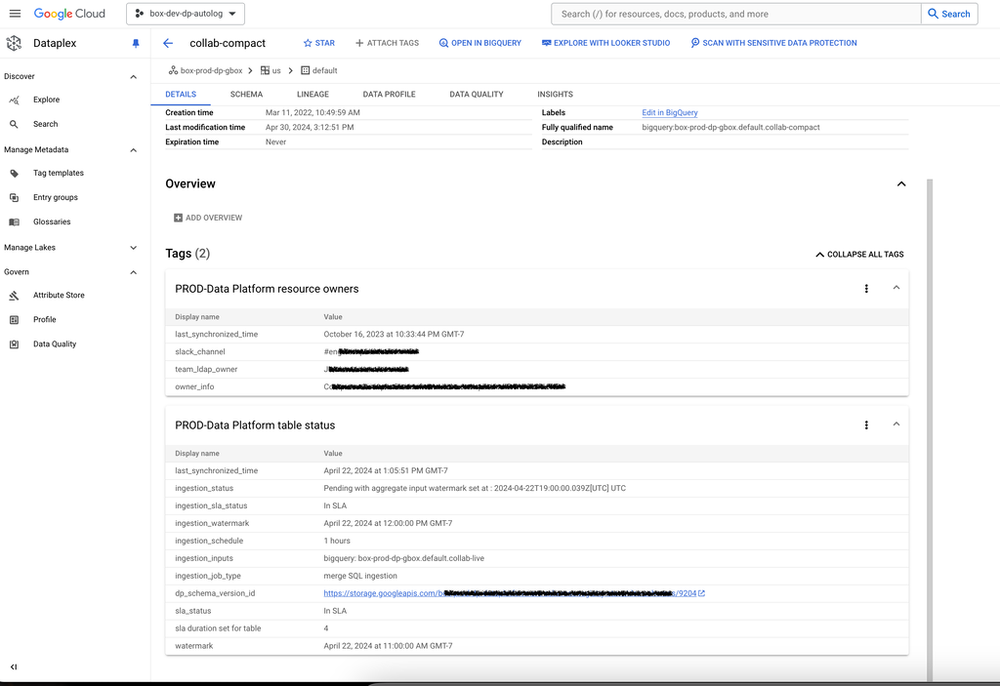
2. Comprehensive data observability via data lineage and custom metadata tags
Box.Inc leveraged Dataplex’s table-level operational metadata tags combined with powerful Data Lineage APIs to achieve end-to-end data observability for all BigQuery ingestion pipelines. By automating metadata tag updates and leveraging data lineage APIs, we ensure critical metadata, such as ingestion watermarks and pipeline status, are accurately captured, empowering data engineers and software developers with end-to-end visibility and traceability of data pipelines. Visualizing the data lineage tracking from sources to targets enabled faster impact analysis, facilitating proactive issue detection and faster resolution, and minimizing downtime.
Further, we are working on ingesting streaming ingestion job-lineage events by calling lineage API from our in-house job scheduler.
3. Strengthened security posture through classification framework and fine-grained access control
Implementing a comprehensive data classification framework enabled domain owners to reliably identify, classify, and protect sensitive data fields. With predefined categories like public, internal, restricted, and customer, the framework provided a foundation for strict fine-grained access control, promoting zero-trust policies. Furthermore, integration with Google Cloud’s Data Loss Prevention (DLP) fortified us against data loss and unintended exposure of sensitive information. DLP acts as a safeguard, minimizing false positives. If less sensitive data is flagged as highly sensitive by DLP, we promptly escalate its classification for enhanced protection.
Architecture for classification framework

Leveraging data catalog metadata tags at the column level streamlines data discovery, ensuring efficient management and protection of sensitive data. We performed holistic, real-time monitoring of data access patterns, facilitating identification of unauthorized user access and enforcing adherence to policy requirements across the data platform.
These are sample table- and column-level tags that we created to expose data classification and sensitivity labeling:


Conclusion
In short, Dataplex has been instrumental in transforming our data platform into a secure, efficient, and scalable data ecosystem. With a focus on data governance, discovery, observability, and security compliance, we are equipped to meet the challenges of data management in the digital age. Dataplex empowers our teams to unlock the full potential of data and drive Box.Inc's continued growth and innovation.